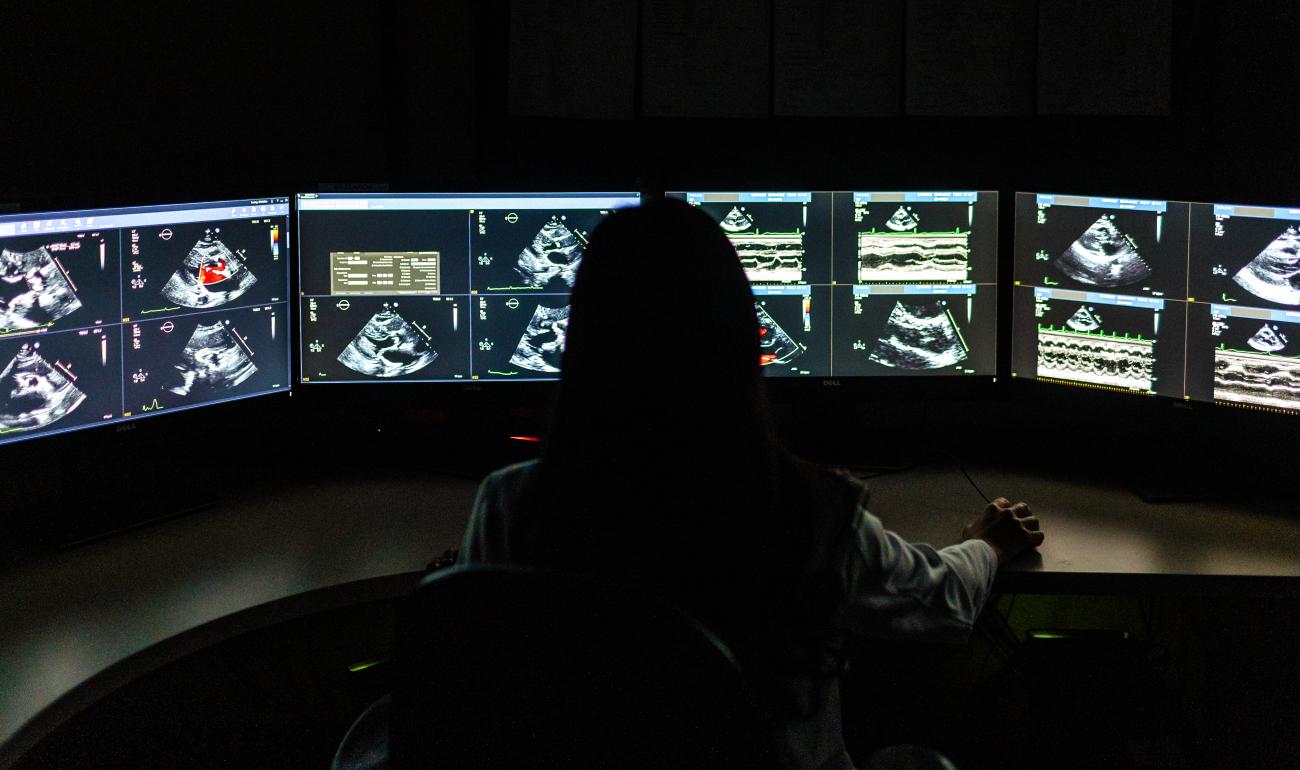
Evidence indicates that AI could enhance the timeliness of heart failure diagnostics using point-of-care ultrasounds.
Researchers have confirmed that artificial intelligence (AI) software has the potential to enhance heart failure diagnostics. The study, led by Vancouver Coastal Health Research Institute researcher Dr. Christina Luong, shows that the machine learning tool could be safely used to improve the timeliness of heart failure diagnosis and treatment.
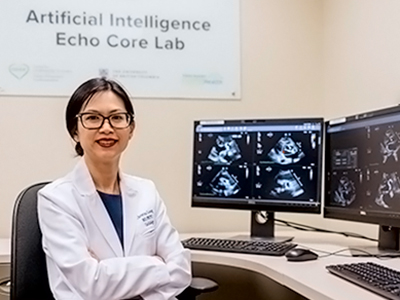
“Wait times for essential echocardiogram diagnostic tests for heart failure presently take up to six months for non-urgent cases, which we would ideally like to see reduced to one month or less,” states Luong. Since around 2014, Luong’s research has focused on the development of machine learning algorithms for imaging diagnostics and research trials that incorporate echocardiography.
“As our population continues to grow and age, AI technologies are helping us relieve some of the pressure on the health care system.”
At around $200,000 each, cart-based echocardiogram machines found in diagnostic imaging labs use sound waves to capture detailed images of blood flow throughout the heart and its many valves. Echocardiograms are the bread-and-butter of diagnostic tests for heart function, as they provide a big picture view of cardiac structure and function, giving insights into the flow of blood inside the heart and throughout the body’s circulatory system, states Luong.
Clinicians use these images to diagnose conditions, such as reduced cardiac pumping function and abnormal relaxation that can decrease blood flow and result in conditions such as pulmonary edema: a build-up of fluid in the lungs that can lead to breathing difficulties. However, the process of analysing images captured by echocardiograms can be time-consuming and requires expertise and experience to reach an accurate clinical diagnosis.
Supercharging heart ultrasound diagnostics with AI
The machine learning algorithm Luong and her team developed and used in her recent study, published in Echo Research & Practice, was assessed for its performance identifying the indicators of disease present in point-of-care ultrasound (POCUS) images. The handheld POCUS devices use sound waves to record video footage of heart function at the bedside on miniature machines, instead of through an appointment with a specialist for an echocardiogram.
"While AI has been validated for use in echocardiogram diagnostics, it has yet to be validated when paired with POCUS scans.”
The research team wanted to know how well their machine learning algorithm measured left ventricular ejection fraction in images captured by both novice and moderately experienced health care workers. Left ventricular ejection fraction is the amount of blood being pumped by the heart within a given time frame — essential information for assessing heart failure and making treatment recommendations.
The machine learning model was previously trained to identify normal and abnormal left ventricular ejection fraction using 2,920 videos from 2,127 patients. While it sifts through video footage, the AI’s assessments are made by video segments, frame by frame, states Luong.
“The sooner a patient receives diagnostic testing, the sooner they can start the recommended treatment.”
For the study, two nurse practitioners with little to no experience using POCUS and seven physicians with moderate POCUS training performed POCUS scans on 138 adult patient volunteers recruited from a heart failure clinic in Vancouver. The 1,257 videos of the patient scans were analyzed by the research team’s machine learning algorithm and an expert echocardiographer.
Luong and her team found a high degree of correlation — around 80 per cent similarity between the AI and expert’s estimation of left ventricular ejection fraction for cardiac POCUS images of heart failure patients. The performance of the model was preserved even when accounting for the scanners with lower levels of POCUS experience.
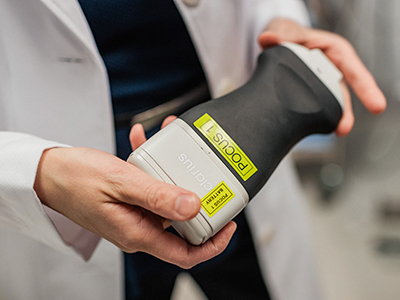
However, the AI algorithm was less accurate in cases of poor image quality or when a patient had an irregular heartbeat, also called atrial fibrillation, which the research team concluded should be considered in potential clinical applications of this type of machine learning model.
“Instead of an expert echocardiographer needing to stop and assess individual frames of cardiac ultrasound scans, machine learning models integrated into clinical workflows can highlight features in the scans and perform tasks, such as taking measurements for the echocardiographer, saving them countless hours of work,” says Luong.
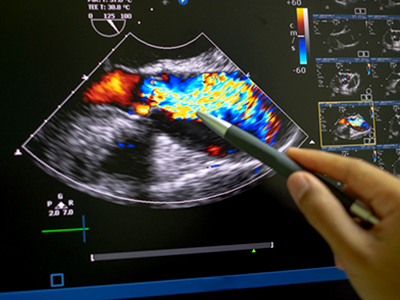
“We are still waiting for larger studies to further validate the use of machine learning in POCUS scans of left ventricular ejection fraction,” says Luong. “The AI space is evolving quickly, which is why we also anticipate its use in many other facets of heart care, including in the prediction of clinical outcomes based on diagnostics.”