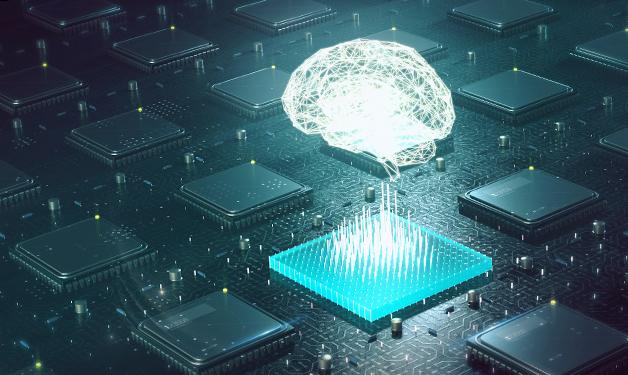
Our expert explains how advances in software technology could change the face of pathology and other clinical practices.
Innovations in artificial intelligence and deep learning are pushing technology closer to being able to perform complex clinical skills, such as diagnosing cancers. Neuropathologist and Vancouver Coastal Health Research Institute scientist, Dr. Stephen Yip, is at the forefront of developments in this area. He explains how deep learning is being used now and its potential impact in the future.
Q: What is deep learning?
A: Deep learning, also known as deep neural networks, is a subset of artificial intelligence (AI), which is the pursuit of simulating human intelligence using machine technology. Deep learning is a multi-layered learning system that, similar to the human brain, can be taught to perform certain functions with the help of training data.
Q: How is it used in pathology?
A: In pathology, we use deep learning to identify disease. We input hundreds, if not hundreds of thousands, of annotated images of diseased and healthy tissue samples into a deep neural network. This teaches its neurons—multiple information detection layers that are able to identify features such as shapes, sizes and colours in an image—how to differentiate between healthy and diseased cells. In a sense, deep learning and clinicians are trained in similar ways—through repetition, filtering information and exposure to many different data sets or images.
Q: How is deep learning applied in clinical practices?
A: Deep learning is currently being researched for use mostly in pathology—disease detection using tissue and bodily fluid samples—and radiology—the use of medical imaging to diagnose disease. This is because both of these diagnostic approaches are highly image-based. In terms of pathology, deep learning, and particularly convolutional neural networks (CNNs), is used to recognize unique features in images taken through microscopes. However, the success of this approach requires a large number of images—often thousands—that are annotated by clinicians to train the algorithm to recognize if part of an image contains, for example, cancerous or non-cancerous cells.
Q: Is it safe for deep learning to be used to identify diseases like cancer? How do we know if the diagnosis is right?
A: Competitions between machines and clinicians have shown that deep learning can match or exceed the accuracy of seasoned experts when it comes to diagnosing diseases such as breast cancer, and in a shorter amount of time. However, this technology is still in the development stages. Deep learning continues to require a lot of human input to make a diagnosis, and it cannot yet replace the work of clinicians in Canada.
Q: How advanced is this technology and what can we expect to see next?
A: A lot of the recent advances have been made possible thanks to graphical processing units (GPUs), which are very well suited to the mathematical operations that are required in deep learning. There is now greater availability of large training sets because more clinicians are uploading and sharing annotated images online in the cloud. Another big advance is the availability of open source code for programming frameworks, which we use to develop industry-specific applications of deep learning, such as disease recognition in pathology.
It used to take around 20-30 minutes to scan and digitize a slide containing a tissue sample, which is then stored and saved in the cloud and on computer servers. The newer generation scanners can accomplish this task in a few minutes and can manage batches of several slides at a time, greatly simplifying and fast tracking the process of digitizing slides. At this rate, the technology shows great promise for improving patient care in the future. We are already seeing signs that deep learning could quickly identify such things as genetic variants and offer long-term prognoses by making connections that would normally be undetected by the human eye.
Q: What about my digital security?
A: There is a natural tendency to be concerned about private information being uploaded to the cloud. However, in this case, there are robust security measures in place to protect patient confidentiality and privacy when working with deep learning. It would not receive approval from regulatory bodies governing privacy and health information in Canada if that was not the case. For example, all of our health data must be stored on Canadian servers and governed by Canadian laws.
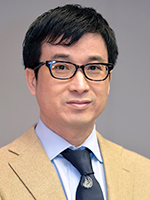